Krokidas P., Karozis S.
Zeolitic-imidazolate frameworks (ZIFs) have the potential to make highly selective membranes, due to their functionalization capabilities that can take place on the molecular level and affect considerably their microscopic properties. However, their application in gas separations is limited due to the lack of knowledge on the modification-properties correlation.
Our approach is based on the premise that the aperture connecting the cages of ZIFs controls the diffusion rate of guest species. In our recent works, we showed that the aperture’s size and flexibility can change, by replacing the organic linker, the metal center or the functional group of the framework. This in turn provides control over the diffusion-based selectivity of a given pair.1,2,3 This knowledge shapes a chemical basis which we used in a novel machine learning (ML) tool towards developing a complete modification/selectivity correlation for ZIFs, as shown schematically in Figure 1. We built numerous new ZIFs of finely discretized aperture sizes, by replacing various units, and simulated the diffusion of guest molecules of varying in size (He, H2 up to n-butane) in these structures, based on force fields developed based on density functional theory (DFT) calculations. The simulations account for the flexibility of the structure, to assess the framework’s response as a function of the gas penetrant’s size. Then we trained a supervised ML model on descriptors with physical (size and weight of the linkers; charge and length of the functional group) and chemical significance (ionic radius of the metal center and information on the metal-nitrogen bond), as well as information on the response of the framework upon a given gas penetrant’s presence.
Overall, our ML tool enables the prediction of the diffusivity of any gas on any ZIF structure and of the diffusivity of any gas in it without the need for molecular level simulations. The only information needed is basic chemical information from DFT calculations and the size of the gas penetrant. This approach can further promote the fast design of process tailored new ZIFs, by providing as input information only the desired diffusivity/selectivity of a given mixture.
1. Krokidas, P. et al. On the Efficient Separation of Gas Mixtures with the Mixed-Linker Zeolitic-Imidazolate Framework-7-8. ACS Appl. Mater. Interfaces 10, 39631–39644 (2018).
2. Krokidas, P., Moncho, S., Brothers, E. N., Castier, M. & Economou, I. G. Tailoring the gas separation efficiency of metal organic framework ZIF-8 through metal substitution: A computational study. Phys. Chem. Chem. Phys. 20, 4879–4892 (2018).
3. Krokidas, P., Moncho, S., Brothers, E. N. & Economou, I. G. Defining New Limits in Gas Separations Using Modified ZIF Systems. ACS Appl. Mater. Interfaces 12, 20536–20547 (2020).
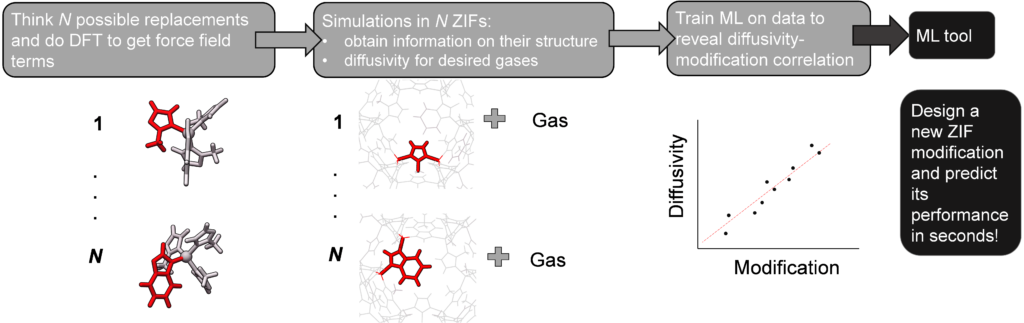
Figure 1. Outline of our ML model for the fast design of top-performing ZIFs with desired performance.